Precision-Recall curve versus ROC curve for imbalanced datasets
- Nate Puangpanbut
- Jan 12, 2024
- 1 min read
Updated: Feb 13, 2024
In Binary Classification, we usually use the ROC curve to evaluate performance of our model, but sometimes it will be misleading especially with imbalanced dataset. This paper shows the benefit of using the Precision-Recall curve instead of the ROC curve that will help us understand our model performance for any imbalanced dataset better.
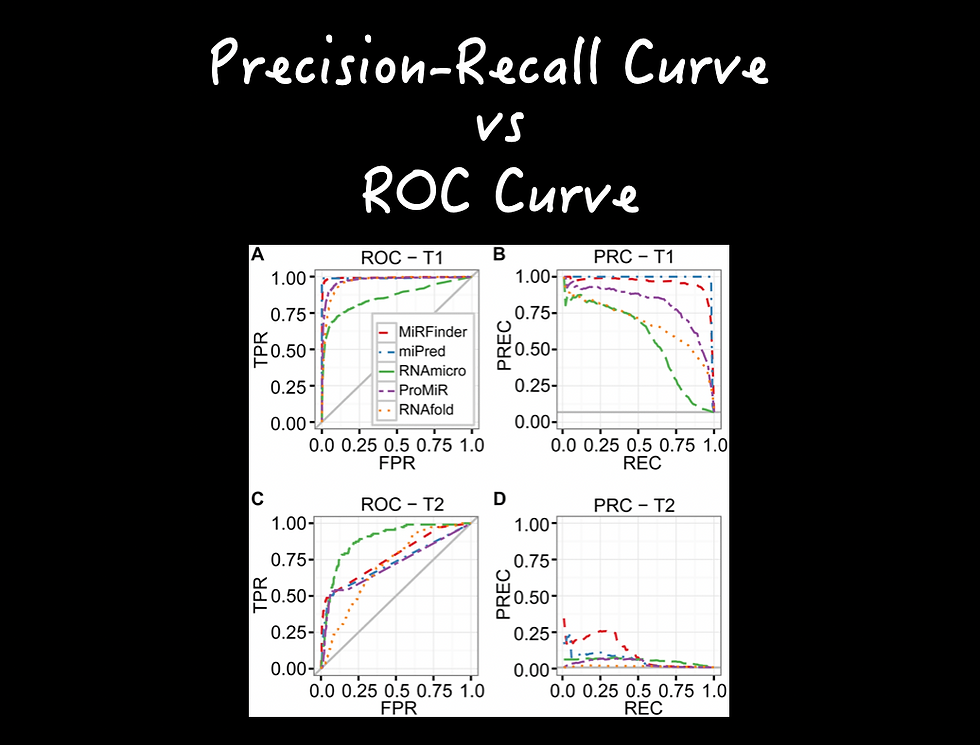
Source
Summary
ROC is a popular and strong measure to evaluate the performance of binary classifiers. However, it requires special caution when used with imbalanced datasets. CROC, CC, and PRC have been suggested as alternatives to ROC, but are less frequently used. This study shows the differences between the various measures from several perspectives. Only PRC changes with the ratio of positives and negatives.
With the rapid expansion of technology, the number of studies with machine leaning methods will likely increase. The analysis suggests that the majority of such studies work with imbalanced datasets and use ROC as their main performance evaluation method. Unlike ROC plots, PRC plots express the susceptibility of classifiers to imbalanced datasets with clear visual cues and allow for an accurate and intuitive interpretation of practical classifier performance. The results strongly recommend PRC plots as the most informative visual analysis tool.
Comentarios